When to use click attribution or Marketing Mix Modeling (MMM) for campaign measurement
Should you throw your ad platform reporting out of the window and migrate to MMM? We cover how two different types of attribution work and how to get the best out of both worlds.
The death of click-based attribution?
MMM (Marketing Mix Modeling) has been picking up steam. This week, even Google launched their MMM solution. There’s been a talk of “the death of click-based attribution” and “a new post-cookie world”. And, although we marketers are not short of dramatic statements, we are short on how these forms of attribution actually work.
That’s why I’ve brought Pranav, founder of Paramark, a platform that includes both MMM and Incrementality testing, to explain how MMM works and when it should be best used. Paramark and Pranav have analysed $100M+ in marketing spend in the last year across B2B, B2C, and Fintech customers. And, as a disclaimer, I’m on Paramark’s advisory council to help them with both Product and GTM.
Defining what we mean by “attribution”
Measuring cause and effect
Attribution is the practice of connecting your marketing activities to business results. The goal is to deduce what was the impact (on revenue, on new sales, on user activity) from your marketing activity.
In principle, attribution is what enables you to pinpoint where to allocate resources, what activities to double down on and what to stop.
The different types of attribution
Attribution is over a century old. You can find Claude C. Hopkins covering how to measure advertising results with voucher codes in his seminal book back in 1923. But, attribution has evolved during our digital era and there are now many, many ways of attributing results to advertising campaigns:
1. Vouchers
It’s 100 years old, but still used all over on OOH as you will notice in any trip on the London tube. It works by including a campaign-exclusive voucher code in the advertising. It enables you to attribute results once consumers redeem the code.
2. Post-view
Less and less measurable due to the growth of privacy features and regulations. Traditionally used by ad platforms to understand which users viewed an ad (but not clicked). Done either by user identification (when both the view and conversion occur in ad platform-tracked environments) or creative wrapping.
3. Post-click
The most prevalent form of attribution is to measure the users that clicked on a certain URL and then went on to convert. This is done by prepending queries in the URL such as UTMs or ad platform click IDs.
4. Zero party data (aka HDYHAU, attribution survey)
Refers to asking users where they heard about you, usually in a form when they sign up or first become a lead. An easy to implement attribution form that is guaranteed to deliver value.
5. Marketing Mix Modeling (MMM)
Employing econometrics, MMM uses aggregated statistics to find the correlation between marketing activities and business outcomes. Can be executed in-house by your data team, with a research agency or with a tool.
We’ll be focusing on the most-prevalent form of attribution (click based) and its new alternative (MMM) for this piece.
Deep diving into click attribution
Connecting clicks and events with URL queries
Click-based attribution connects a click to a marketing campaign to a conversion event that happens on a digital property you own (website, app).
They do that by appending “queries” to the URL that will include information that’s relevant to the marketing campaign. For example, let’s assess the following URL:
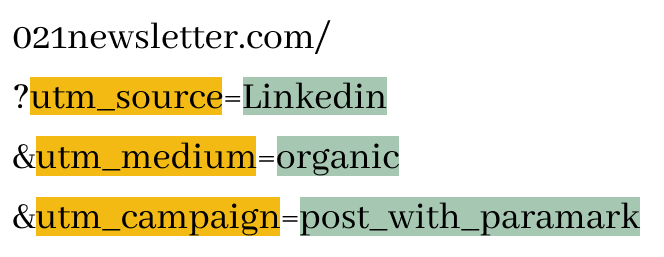
I’ll post this article on Linkedin with the following URL. When you click on it, you’ll land on my Google Analytics property (021newsletter.com). Even though you’ll be on the homepage, like many other users, I will know from the URL queries where you’ve come from.
On paper, if you go on to subscribe to my newsletter, even if you’ve returned to my site via a different source, we can measure that you first entered the site through an Linkedin post.
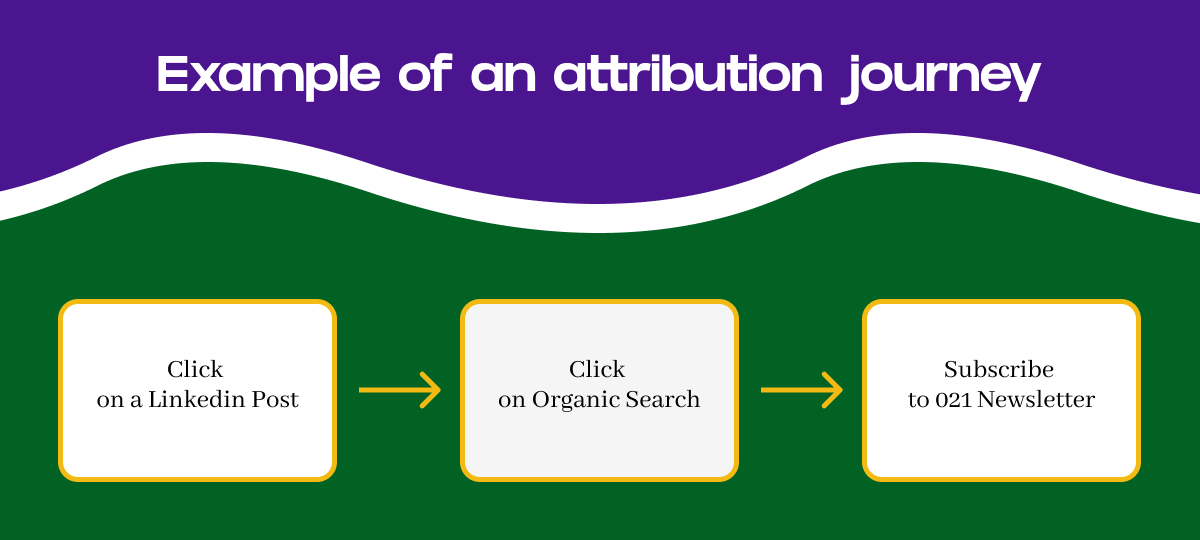
On click based attribution, you can also specify:
Which touch should get the credit: In the example above, a first-click attribution would give the full credit to Linkedin, a last-touch attribution would give the full credit to Google and a multi-touch model would split the conversion between the two platforms.
Lookback window: How long the period of time after which an ad is clicked that a conversion can be attributed to the ad. For example, if your lookback window is 10 days but the Linkedin click was 15 days ago, then the full credit will go to Google regardless if your model is first-click.
Ubiquitous in tracking
Although most ad platforms today use a mix of attribution models and sources, clicks and URL queries play a vital role. Most platforms have their own “unique queries” which are used to measure click attribution. You can find click based attribution being used all over the web:
Ads: gclid, gbid, maid etc.
Lead tracking: hsa_, etc.
Website Analytics: utms, t, etc.
One of the reasons that click-based attribution is less and less accurate is the demise of URL queries. New privacy features, like by Apple’s, are removing these values from URLs.
Past, present and future gaps in click attribution
In a lot of cases, the user doesn’t convert during the same session where they clicked on the ad. Meaning, they click on an ad, land on the page, but go on to purchase (or perform your defined conversion event) at a later time or date.
In this case, the attribution platform must identify who this user is in order to stitch the two sessions together. And that’s where we’re facing issues. In most of these situations, platforms are unable to do this due to a variety of factors:
Cross-device: User clicks on mobile web but converts on desktop web
In-app: User clicks on mobile app (e.g. Instagram ad) but converts on mobile web
Consent: User doesn’t consent to being tracked
Private Browser: User visits page via a private browser such as Safari privacy features on or Brave
Time: Cookies expire before the conversion event
Media consumption: many users will consume your ads on social/video feeds but search and click through from search channels, leaving you to think that search drove the click
There’s also an assumption that because event A (click) happened right before event B (conversion), that event A caused event B. This may or may not be true and click attribution doesn’t understand the uncertainty implied in this statement.
Now, now: let’s dive into MMM
What is MMM?
Marketing Mix Modeling (MMM) is a type of statistical analysis that provides valuable insights into the effects of marketing activities on business outcomes (traffic, sales, etc.). MMM gives marketers a comprehensive view of which channels are driving incremental returns for the business.
It works by analysing trends in your marketing inputs and outputs. When reach or impressions on a channel or campaign goes up, do you see a corresponding increase in your business outcomes? Vice versa, when impressions decline, do you see a decrease in those same outcomes? If yes, that’s an indication of a correlation.
The benefits of MMM, in contrast to click attribution, are:
Channel agnostic: works for all types of channels (OOH, TV, Video, Social, Search, Influencer, and everything in between), even if the channel doesn’t drive “clicks”. This enables you to compare performance from very different channels as “apples and apples”.
Objective correlation: identifies the objective correlation of each channel and strategy with business KPIs, instead of assuming correlation in the event stream data. Just because the user clicked on a campaign and converted, it does not mean they converted because they clicked.
Privacy friendly: addresses data loss due to privacy changes by not relying on device or identity resolution, and cookie consent.
How do you set up MMM?
There are a variety of open source solutions, consulting vendors, and now software solutions for MMM. Regardless of your choice, here’s how we recommend you go about setting up MMM for your business.
Step 1: Inputs & outputs
First, pick the right target (aka output) metric(s) that you want to optimise. This is different for every business. Here’s a post on how to do just that.
Second, collect all your marketing input data. This includes impressions and costs across every channel for the last couple of years, aggregated on a daily basis.
Step 2: Validation & modeling
As we all know, “garbage in, garbage out” is a reality with any data project. It’s true for MMM as well. So, start with validating your data to make sure you’re not seeing any strange patterns.
E.g. if you changed definitions of your output metric halfway through the period you’re analysing which caused a steep drop in your metic. In this case, either only look at the period after the definition change or find a way to generate the metric with the new definition for the historical time period.
Then, work with your data scientist to run this data through any open source package (e.g. LightweightMMM from Google) or work with a consulting / software solution.
Step 3: Analysis & refresh
Now you’re ready to analyse the results and make recommendations to your team and execs. The type of insights you get are:
Break out of your output metric (e.g. Sales) into baseline and incremental
Cost of incremental output metric (e.g. Sales) - aggregate and by channel/strategy
Diminishing returns by channel and strategy - i.e. how does sales contribution change as you increase or decrease your spend
You can now refresh your model every month or quarter as you wish.
When is MMM not suitable for brands?
MMM is a correlational analysis and requires sufficient data to be effective and valuable. Here are some basic criteria to help you decide whether it will work for you:
You have at least twelve months of daily data for inputs and output metrics.
You have sufficient variability in your output metric data (think at least 10s or 100s of output metric on any given day).
You have at least three or more channels to analyse. While this is not a strict criteria, the value of insights from MMM is diminished when you’re only working with one or two channels.
The role of incrementality measurement
Incrementality is the true topic of concern in attribution. I.e. does your marketing drive net new business outcomes that wouldn’t have been possible without that marketing. MMM helps you estimate that incremental return - both at a high level (across your entire marketing spend) and at a granular channel and campaign level.
This becomes the foundation for your ROI calculations, and helps you understand which channels are worth doubling down on and where you need more work before you can invest more money.
Incrementality testing that is the only true way of understanding the causal link between marketing and sales outcomes.
Paramark automates this analysis by connecting directly into your data warehouse and other marketing platforms, refreshing MMM on a weekly or monthly basis, and generating dashboards with actionable insights whenever you need them.
Beware though, that correlation is not causation. There’s another related practice of incrementality testing that is the only true way of understanding the causal link between marketing and sales outcomes.
When to use MMM or click attribution?
Planning, forecasting, budgeting: MMM
By answering the question of incrementality, MMM is well suited to help you throughout your planning cycle. From annual planning, to quarterly forecasting, and monthly performance tracking, MMM works very well.
It helps you understand which channels and campaigns are beating your goals, and how to reallocate budget to further improve your business outcomes.
Measuring awareness campaigns: MMM
Channels and campaigns that are focused on top of funnel or awareness (e.g. TV, Video, OOH, Influencer) and aren’t necessarily driving clicks will still show up in your MMM analysis. This isn’t the case for click attribution, which will use “view” metrics like Impressions and Video Completion Rate to measure their impact.
Although there are new metrics to measure awareness campaigns in specific, like Attention metrics, MMM is better suited to understand how incremental each awareness strategy is.
Campaign optimisation: Click
When it comes to optimising ad groups, ad copy, creatives, targeting and placements within a campaign, click-based attribution will provide you the most granular and up to date information.
Same-session attribution is still “as accurate as it can be” for click-based measurement. Therefore, you can rely it to measure:
Engagement on site: if they go on to spend a certain amount of time on the site or visit an X number of pages
Top of funnel events: if they download a leads asset, subscribe to a newsletter or perform another low effort action.
Alongside that, you can also leverage ad platform engagement metrics (CTR, CVR) to optimize your campaigns and creatives mid-flight.
The future of attribution is triangulated
One of the biggest advantages of digital campaigns are the fast signals that we get from them, thanks to mid-flight event tracking and ad platform metrics. This data holds a lot of value, that goes from understanding what audiences are interested in your brand to identifying the best performing creatives.
On the other hand, click based attribution has gotten less and less accurate with the tendency of the picture to still get murkier. If you have a full-funnel marketing approach and you’re only looking into click attribution currently, there’s no doubt you’re making decisions on incorrect data.
The best approach to attribution is triangulation. Where there’s no “one source of truth” but each data source provides a unique value.
Learn more about attribution and marketing data
If you want to learn more about attribution and marketing data, you’ll be happy to know that both
and I write on the topic. Pranav writes about the science of marketing on the Paramark blog.I cover marketing data and how to leverage insights for growth in this very 021 Newsletter. So if you’re interested in getting more out of your marketing data, you know what to do:
This is a great article that touches on key attribution and marketing mix optimization topics.
"The future of attribution is triangulated" says it all. As systems become more complex and harder to track, having your attribution sources developed will become key in effectively allocating budgets and keep growing!
Definitely worth the read